

- #Nvidia cuda toolkit driver 5.0 install
- #Nvidia cuda toolkit driver 5.0 code
- #Nvidia cuda toolkit driver 5.0 Pc
Sudo apt-get install linux-headers-$(uname -r) Then install linux-headers package for that kernel version: Verify the System has the Correct Kernel Headers and Development Packages Installed Note: gcc is required for development using CUDA Toolkit, not required for running CUDA applications.Ĥ. Native Linux Distribution Support in CUDA 11.2" in CUDA Installation guide. Note: Please refer to OS support matrix "Table 1. Verify OS version uname -m & cat /etc/*release Note: update-pciids fetches the current version of the pci.ids file from the primary distribution site and installs it.Ģ. Subsystem: NVIDIA Corporation Quadro RTX 6000 # update-pciidsĭownloaded daily snapshot dated 03:15:02ġ7:00.0 VGA compatible controller : NVIDIA Corporation TU102GL (rev a1) (prog-if 00 ) I apologize for the misleading question.This article records each step for installing CUDA Toolkit and NVIDIA Driver on Ubuntu by following CUDA installation guide.ġ. However, I am a beginner, so I'd better not advance hypotheses riskly. This must be explained by the fact that the Profiler uses much more memory to trace the timeline in this modality, so the limit of the number of streams is lower than in the synchronous modalitiy. I thought that it was a problem of the Profiler besause the application with the two methods runs correctly, and it only stops when I run it from the Profiler in the "Enable concurrent kernels execution" modality to trace the timeline. Instead, it was a problem of memory: simply, in the application that contains both the CUDA version and the OpenCV version of my algorithm, I use a number of streams that is twice the size of that I use in the applications with only one version of the algorithm, and this exceeds the memory capacity of the Profiler.

Well, trying to solve my problem, I experimented that it was not a problem of CUDA Context: applications written using both CUDA and OpenCV are traced well by the Profiler. How can I manage the CUDA Context in order to allow the Profiler to trace the timeline? I think this may be caused by the fact that OpenCV calls should use the same CUDA Context as the rest of the CUDA code. Indeed, it stops working when I mix the two in the same application.
#Nvidia cuda toolkit driver 5.0 code
It creates the timeline correctly both for code written using ONLY OpenCV functions and for code written using ONLY CUDA functions. Unfortunately, CUDA 5.0 NVIDIA Visual Profiler can't trace the timeline of my application if I enable the feature: "Enable concurrent kernels profiling".
#Nvidia cuda toolkit driver 5.0 Pc
For this reason, I built (succesfully) the source code of OpenCV 2.4.2 with this Toolkit (5.0) installed on my pc (this was suggested to me on the OpenCV blog), and I'm able to compile and execute correctly my application with concurrent kernels: some of them are invoked by functions of the module OpenCV_GPU and others are kernels I directly wrote in CUDA. I'm using CUDA Toolkit 5.0 because I need to use the new feature of the NVIDIA Visual Profiler of this Toolkit that allows to view in the timeline concurrent kernels executed asynchronously (this is not possible with the CUDA Toolkit 4.2).
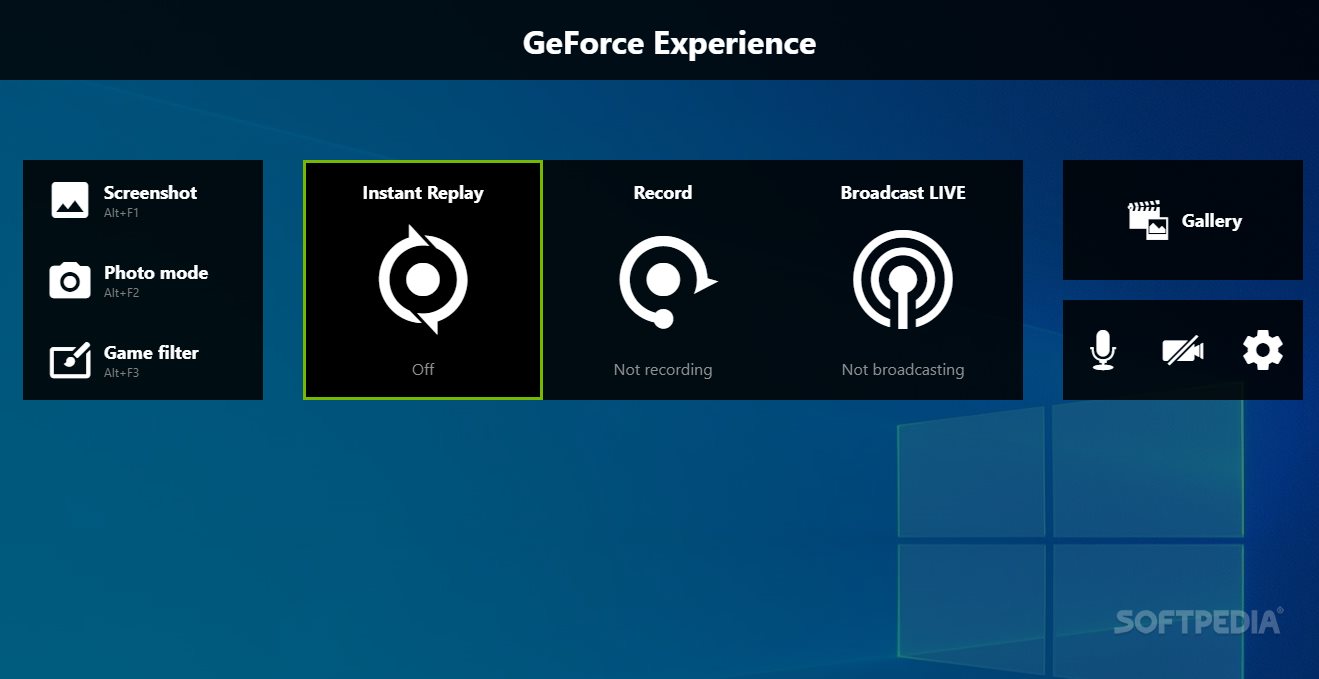
Compiler: Visual Studio 2010 Professionalĭevice: GeForce GTX 680 or GeForce GT 650M
